Dear Reader,
In the face of significant global biodiversity loss, assessments highlight that Asia is the most underperforming continent in achieving global biodiversity targets due to factors such as rapid deforestation and cash crop plantations, construction of mega infrastructure projects, urbanisation, and unplanned use of natural resources.
As this crisis deepens, technological innovation is stepping up to play a critical role in conservation. A team of experts, primarily from Asia, has developed principles on Artificial Intelligence (AI) for biodiversity conservation, anticipating the growing use of AI in this space. Deep learning, computer vision, environmental DNA (eDNA), and networked sensors are seen as game-changers by global conservation experts. Leading up to last year’s United Nations Biodiversity Conference of the Parties (COP16), AI gained remarkable attention, including initiatives like the first International Summit on Indigenous Communities and AI.
However, as a field that has used digital technologies for decades, practitioners have been cautious in adopting advanced technologies for conservation, warning that technology alone will not solve all biodiversity challenges. In the last few years, across Asia, deep learning in ecological research has shifted focus from purely technical studies to addressing practical issues like land use change, biodiversity conservation, climate change, and societal impacts.
We examine existing and cutting-edge technologies in ecological research and conservation, the limitations and risks of data, and some new developments in funding models.
Spotlight: The Cali Fund
At COP16 in November 2024, we saw strides towards implementing biodiversity targets set in the 2022 COP15 Global Biodiversity Framework (KMGBF). Notably, we saw the creation of the Cali Fund, which focuses on sharing the benefits and profits from the use of Digital Sequence Information (DSI) of genetic resources to develop biotechnology products. Under the Cali Fund, large companies benefiting commercially from DSI use “should” contribute 1% of their revenue into a fund allocated to support the self-determined priorities of indigenous groups in implementing the KMGBF. However, conservationists are sceptical - there is no unified enforcement mechanism for contributions, and coordination is needed for open access to data, database interoperability, and better benefit-sharing outcomes.
June Rubis, a conservationist and part of the Global Council, ICCA Consortium, and International Indigenous Forum for Biodiversity, who was in Cali during the negotiations, told us that while the Fund is an important acknowledgement of the contributions of Indigenous Peoples, Afro-descendants, local communities, and Global South countries to biodiversity conservation, true reciprocity requires more than financial contributions — it demands the recognition and support of Indigenous governance systems and rights.
“Mechanisms like the Cali Fund must ensure that benefits are not only equitably shared but also directly controlled by Indigenous Peoples and local communities, respecting their self-determination and traditional knowledge. Without enforceable commitments and a rights-based approach, the promise of equitable benefit-sharing risks being unmet.”
Curated Reads
In this section, we look at use cases for AI in conservation, ethical tensions that arise, and the data question. Find definitions of some key terms in our climate glossary.
GeoAI - mapping and monitoring for conservation
After our last issue, we’re revisiting GeoAI to highlight its critical use for biodiversity conservation. In this article on deep learning and satellite remote sensing, the authors experiment with Convolutional Neural Networks (CNN) and Recurrent Neural Networks (RNN) for spatial and temporal data, respectively, enabling tasks like mapping invasive species, monitoring vegetation, and counting animals like elephants and whales.
However, these tasks require high-quality labelled datasets and computational resources, usually inaccessible to Global South institutions. Other barriers to adopting technology include algorithm complexity, and environmental costs of computation, underscoring the need for sustainable practices and better data access in the field.
The emergence of bioacoustics and AI
Bioacoustics serves as a tool to document the changes to an ecosystem over time; recording biodiversity loss, monitoring species population, and tracking migratory patterns. It can be used to distinguish between similar species as well, proving useful in tackling the songbird trade crisis in East and Southeast Asia that threatens many species with extinction. A new deep learning-based bioacoustic tool can identify 15 morphologically similar White-eye species using bird vocalisation data to aid citizen scientists and law enforcement officials.
AI tools for marine ecosystems
To protect marine environments, innovative, noninvasive monitoring methods are essential. Traditional approaches often harm ecosystems and rely on field expertise. This review highlights the use of environmental DNA (organic genetic material like skin cells), metabarcoding, and high-resolution underwater imaging, which provide more precise and automated ways to monitor biodiversity.
Researchers compared machine learning (CatBoost) and multivariate linear models to monitor the Indo-Pacific eel in central Vietnam. The eel (Anguilla marmorata), vital for global ecosystems and fisheries, faces threats from overfishing and habitat loss, necessitating conservation efforts. They found that CatBoost achieved high accuracy and effectively estimated eel length, outperforming traditional models. These findings underscore the potential of ML tools in mapping species distribution and growth, providing valuable insights for conserving marine biodiversity and their habitats.
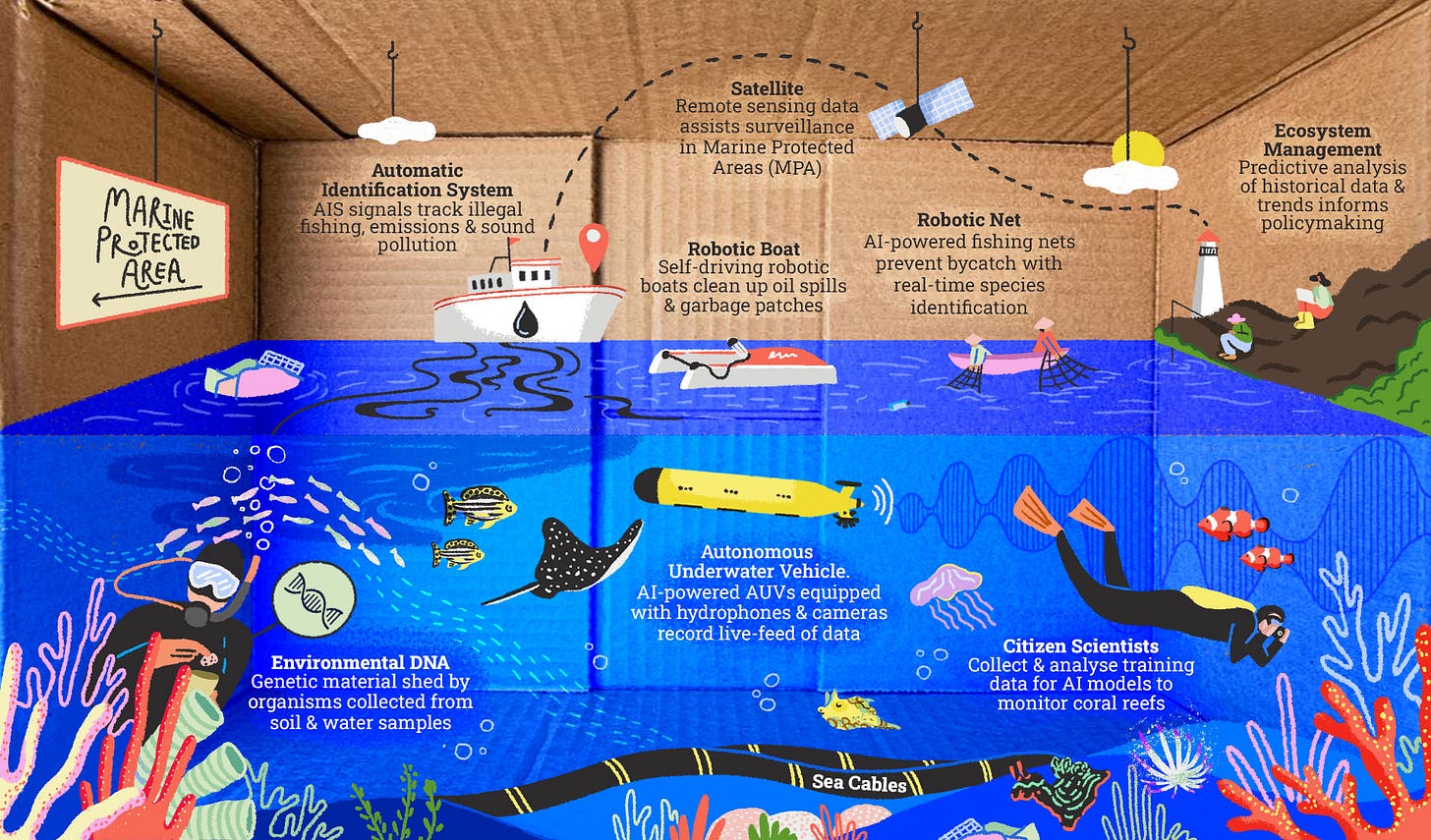
Sounds great, but what’s the catch?
The use of AI/ML is slowly but steadily increasing in conservation, but like other sectors, it is accompanied by risks and ethical considerations. In the case of remote sensing, York et al unpack the discursive landscape of justice and ethics in conservation technology, addressing tensions between anthropocentric and multi-species ideas of justice.
Potential risks to marginalised groups include oppressive surveillance and criminalisation of marginalised groups who might be reliant on forest produce for livelihoods. For instance, in a recent study of camera traps and drones in the Corbett Tiger Reserve in India, researchers found that cameras monitoring dry stream beds to track elephants and tigers had inadvertently captured a semi-nude woman in the area, which was later circulated on social media by temporary forest personnel.
Narratives on multi-species ideas of justice tend to focus on how technology-driven real-time monitoring can prevent illegal wildlife trade activities. The fear associated with such surveillance technologies is also considered a positive in some literature and the monitoring itself is framed as an ethical imperative, rather than a risk. However, for conservationists, it can be a dilemma to decide how much data should be shared with law enforcement officials if these technologies also capture the movement of local communities accessing forest produce in protected areas.
Data justice for biodiversity conservation
High-quality data is a critical scaffolding for developing AI applications. But the justice implications of data in conservation remain underexplored, particularly how data shapes representations, decisions, and the distribution of benefits and harms. In their work on data justice in biodiversity conservation, Pritchard et al identify the issues with global biodiversity datasets such as bias towards particular locations which have implications for how data is used and conservation resources are distributed. Those collecting and using the data are also distant from the people and environments represented in the data.
For systems that support people in understanding the use of data, recognising biases and challenging potential harms, they propose a data justice framework for conservation across five elements: data composition (what or who is represented), access and control (who benefits from data), use (how data is analysed), and consequences (outcomes of data-driven decisions).
AI for biodiversity conservation - being responsible
The Global Partnership on AI (GPAI) has put together a seminal report on the intersection of AI and biodiversity. They categorise some key risks from the use of AI:
Impacts risks, where the use of AI does not add any value or is used as a means of greenwashing to distract from fundamental changes to business models;
Social risks, when AI is deployed in languages local communities do not understand or is used to monitor environmental defenders; and
Environmental risks, in how AI entrenches unsustainable business models and drives e-commerce consumerism, increasing the demand for resources and extraction with impacts on biodiversity.
Some of the key recommendations in the report include:
Establish a community-data dialogue to identify sensitive data and explore tools like federated learning, edge computing and privacy-enhancing technologies for data use and sharing while respecting community data rights.
Encourage philanthropic foundations to adopt an open funding model, actively supporting applications from the Global South.
AI-for-biodiversity projects should explore offering services to the private sector as the market for biodiversity-positive business models grows.
On our radar: Interspecies Money
The lack of funding for conservation is a persistent challenge, even preventing clear pathways forward at COP16. This has led to some creative funding instruments from the private sector. For example, what if we could expand our definition of ‘money’ beyond human transactions to include how different species might contribute to or affect each other's well-being and ecosystems? The concept of Interspecies Money emerged out of this very question for Jonathan Ledgard and his company, Tehanu, becoming the first to operationalise the concept and make an ‘interspecies money’ transfer in 2024. Tehanu’s AI model assesses the needs of gorillas, providing conservation-related advice to agents using it, who receive mobile payments based on pre-determined values of the species when conservation targets are met.
Many of these interoperable systems and transfers are automated, leading sceptics to ask — who ascribes this value and how can interspecies exchange even be commodified? In this pre-print, researchers document how a digital stewardship and reward system for biodiversity conservation was implemented in a game reserve in South Africa. One of the tensions they highlight is in the often arbitrary nature of allocating monetary value to species protection - for example, if the returns from poaching or illegal trade are higher, how does one incentivise the protection of a particular species?
Around the web
Global Ecosystems Atlas: Launched at COP16, the Atlas is the first comprehensive map of the world’s ecosystems.
WildMe: Explore the impact of crowd-sourced image databases on wildlife conservation in this article documenting databases made by conservationists and citizen scientists.
Earth.fm: Immerse in a library of natural soundscapes from field recordists around the world.
Wings for Wildlife: In this compendium of 15 case studies from diverse ecosystems across India, researchers evaluate the integration of drones in conservation practices.
In this month’s podcast, listen to Eleanor Slade and VV Robin, both conservationists working across Asia, talk about how technology has improved human engagement with nature for the better but why challenges around data and financing mean we still have a long way to go in using AI for conservation.
Credits
Research and curation: Dona Mathew, Meredith Stinger, Tammanna Aurora | Illustrations: Nayantara Surendranath | Art Direction: Tammanna Aurora | Layout Design: Shivranjana Rathore
This is an amazing resource. Looking forward to reading the next post!